News Story
UMD Researcher Uses AI to Predict Floods
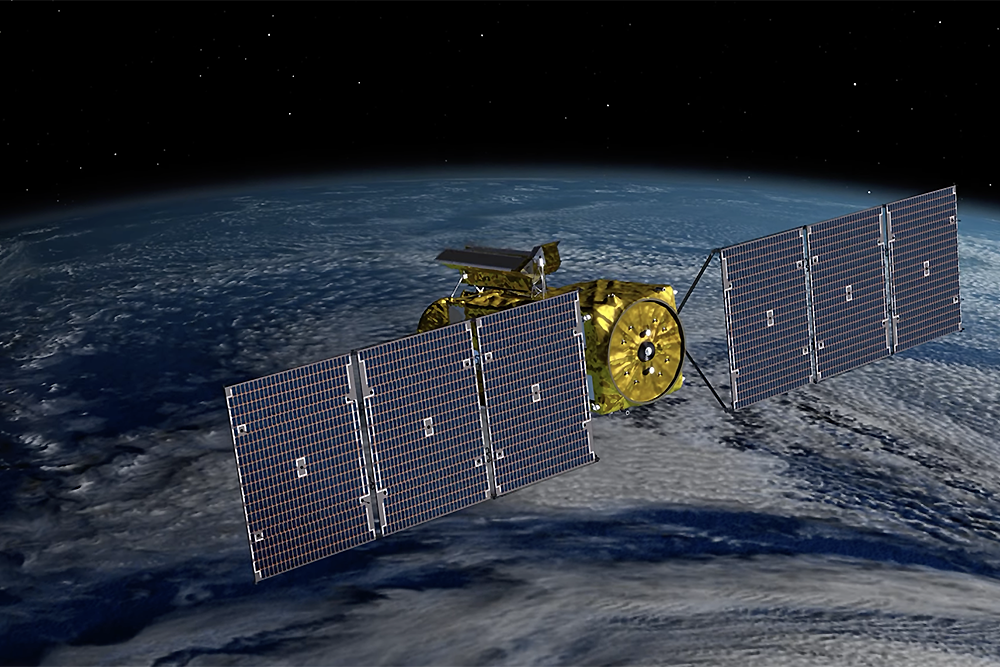
The NASA model, developed by UMD associate professor of civil and environmental engineering Barton Forman, will make use of imagery from commercial and governmental satellites, including the recently-launched U.S.-European Surface Water and Ocean Topography (SWOT) mission mission. [Photo credit: NASA].
Fires have been on the rise in recent years, in many cases because of shifting climate patterns. And where there are fires, there is also an increased risk of flood damage—particularly since intense precipitation is also on the rise.
“We have more fires and they are becoming more extensive.” says Barton Forman, associate professor of civil and environmental engineering at the University of Maryland (UMD). “At the same time, we’re seeing that precipitation events are also becoming more intense. When you take a fire-burned area and couple it with intense precipitation after the fire, you get a really dangerous flood.”
A wide array of stakeholders—from scientists to disaster response agencies to first responders—would benefit from the ability to predict flood events more accurately, particularly those that occur in conjunction with fires. Through a NASA pilot project, Forman has been developing a novel system that aims to provide this level of accuracy–and even train itself to improve.
“The idea is to develop an autonomous, artificially intelligent system that can predict where flood events may occur, with particular relevance to fire-driven change preceding the floods,” Forman said. “The system autonomously collects satellite imagery and uses this imagery to make predictions. It’s also pulling in data from actual events and comparing it to the predictions, so it can adjust itself as needed. And it keeps doing this day after day, in a ‘rinse and repeat’ process that leads to an increasingly refined model.”
Such an approach is made possible by two recent technological trends, Forman said. One is machine learning. The other is the availability of imagery from small, agile commercial satellites.
“In the past, we had a relatively limited amount of imagery that was obtained using NASA satellites,” Forman said. “NASA’s imagery is of a very high quality and it covers a lot of the planet, but it’s usually coming from one monolithic platform with seven or eight sensors, and it can’t see everything at once. Flood events can occur between satellite overpasses, and then we end up without the necessary data.
“But in the commercial arena, they’re building and launching large numbers of smallsats–there are literally hundreds of them in orbit around the Earth. That means we can see the entire globe every day, instead of every few days as was the case in the past. We can also have satellites that can slew or rotate so that they look at different parts of the globe. We can thus capture the information we care most about–the runoff, the floods.”
Space systems, whether commercial or governmental, aren’t the only potential data source that a flood-forecasting AI could use. While the current pilot project, which wraps up this year, focuses specifically on satellite imagery, Forman says the concept could be expanded to include imagery obtained from aircraft, including unmanned aerial vehicles (UAVs).
“Conceptually, what we’re building here is similar to the Internet of Things (IoT), where you have any number of devices that communicate with each other and share data,” Forman said. “What we envisage is like the IoT, but with UAVs, ground based instruments, and space based platforms all communicating through the same computer-based network.”
Together, the various data sources support what is known as a “Bayesian merging process,” which starts with an informed estimate of probability that can be brought into ever-sharper focus as more and more data comes.
For end-users such as disaster recovery and emergency management personnel, that could mean greater lead time as well as more efficient and effective allocation of resources.
“If we think that in four days there will be a big flood event, then we can start moving people away from that watershed and bring in supplies,” Forman said. “We can be better prepared to mount an effective response and save lives.”
Published December 20, 2022